求两个直方图的卷积
两个随机变量 x 和 y 之和的概率分布由各个分布的卷积给出。我在进行数值计算时遇到了一些麻烦。在下面的示例中,x 和 y 是均匀分布的,它们各自的分布近似为直方图。我的推理是,应该对直方图进行卷积以给出 x+y 的分布。
from numpy.random import uniform
from numpy import ceil,convolve,histogram,sqrt
from pylab import hist,plot,show
n = 10**2
x,y = uniform(-0.5,0.5,n),uniform(-0.5,0.5,n)
bins = ceil(sqrt(n))
pdf_x = histogram(x,bins=bins,normed=True)
pdf_y = histogram(y,bins=bins,normed=True)
s = convolve(pdf_x[0],pdf_y[0])
plot(s)
show()
给出以下内容,
换句话说,如预期的那样,是三角形分布。但是,我不知道如何找到 x 值。如果有人能在这里纠正我,我将不胜感激。
The probability distribution of the sum of two random variables, x and y, is given by the convolution of the individual distributions. I'm having some trouble doing this numerically. In the following example, x and y are uniformly distributed, with their respective distributions approximated as histograms. My reasoning says that the histograms should be convoluted to give the distribution of, x+y.
from numpy.random import uniform
from numpy import ceil,convolve,histogram,sqrt
from pylab import hist,plot,show
n = 10**2
x,y = uniform(-0.5,0.5,n),uniform(-0.5,0.5,n)
bins = ceil(sqrt(n))
pdf_x = histogram(x,bins=bins,normed=True)
pdf_y = histogram(y,bins=bins,normed=True)
s = convolve(pdf_x[0],pdf_y[0])
plot(s)
show()
which gives the following,
In other words, a triangular distribution, as expected. However, I have no idea how to find the x-values. I would appreciate it if someone could correct me here.
如果你对这篇内容有疑问,欢迎到本站社区发帖提问 参与讨论,获取更多帮助,或者扫码二维码加入 Web 技术交流群。

绑定邮箱获取回复消息
由于您还没有绑定你的真实邮箱,如果其他用户或者作者回复了您的评论,将不能在第一时间通知您!
发布评论
评论(1)
为了继续前进(朝着更模糊的细节),我进一步调整了您的代码,如下所示:
因此,给出如下图:
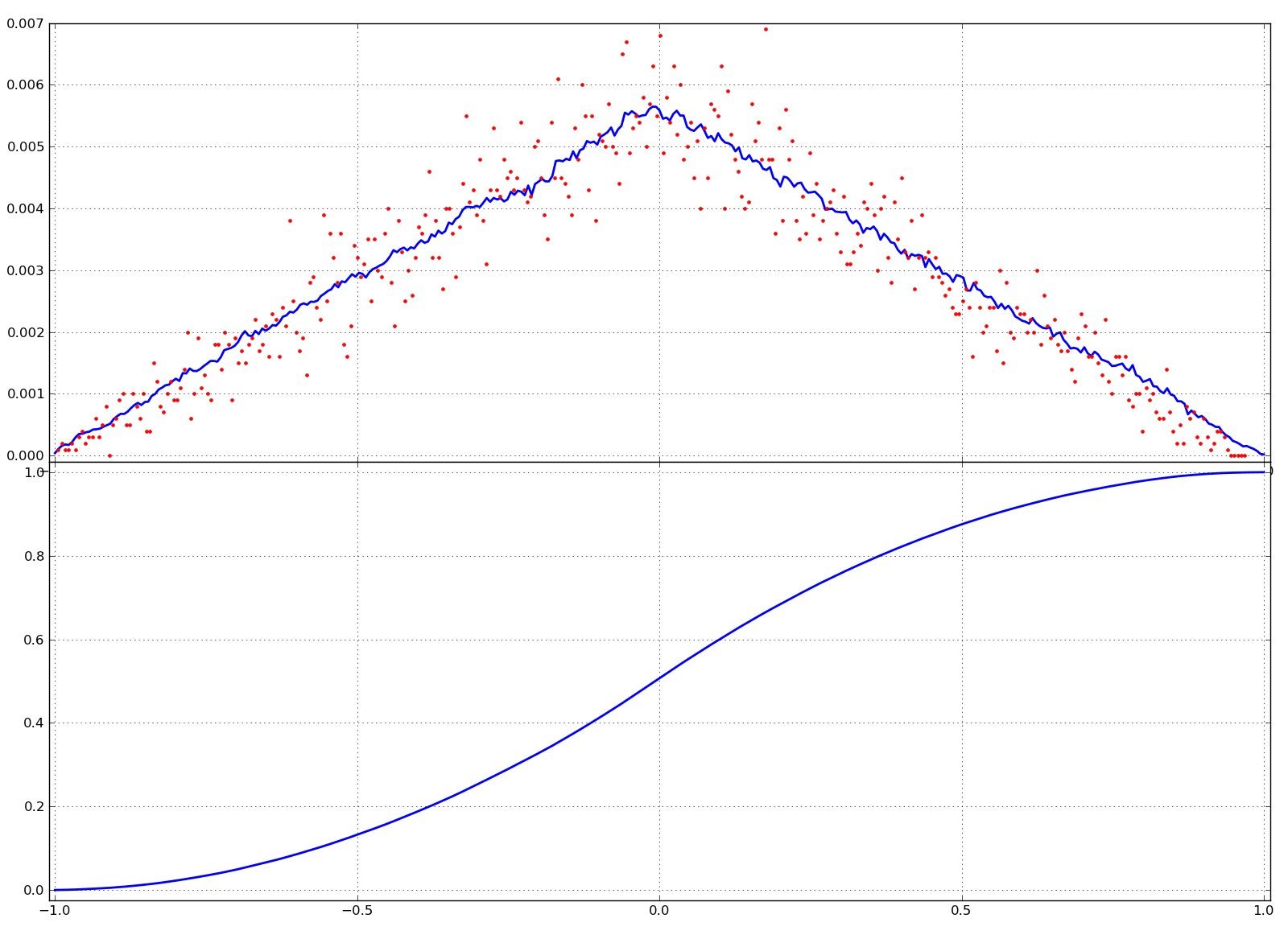
其中上部代表
PDF
(蓝线),它确实看起来很三角形,而模拟(红点)则反映了三角形形状。下半部分代表CDF
,它看起来也很好地遵循预期的S
曲线。In order to still move on (towards more murky details), I further adapted your code like this:
Thus, giving plots something like this:
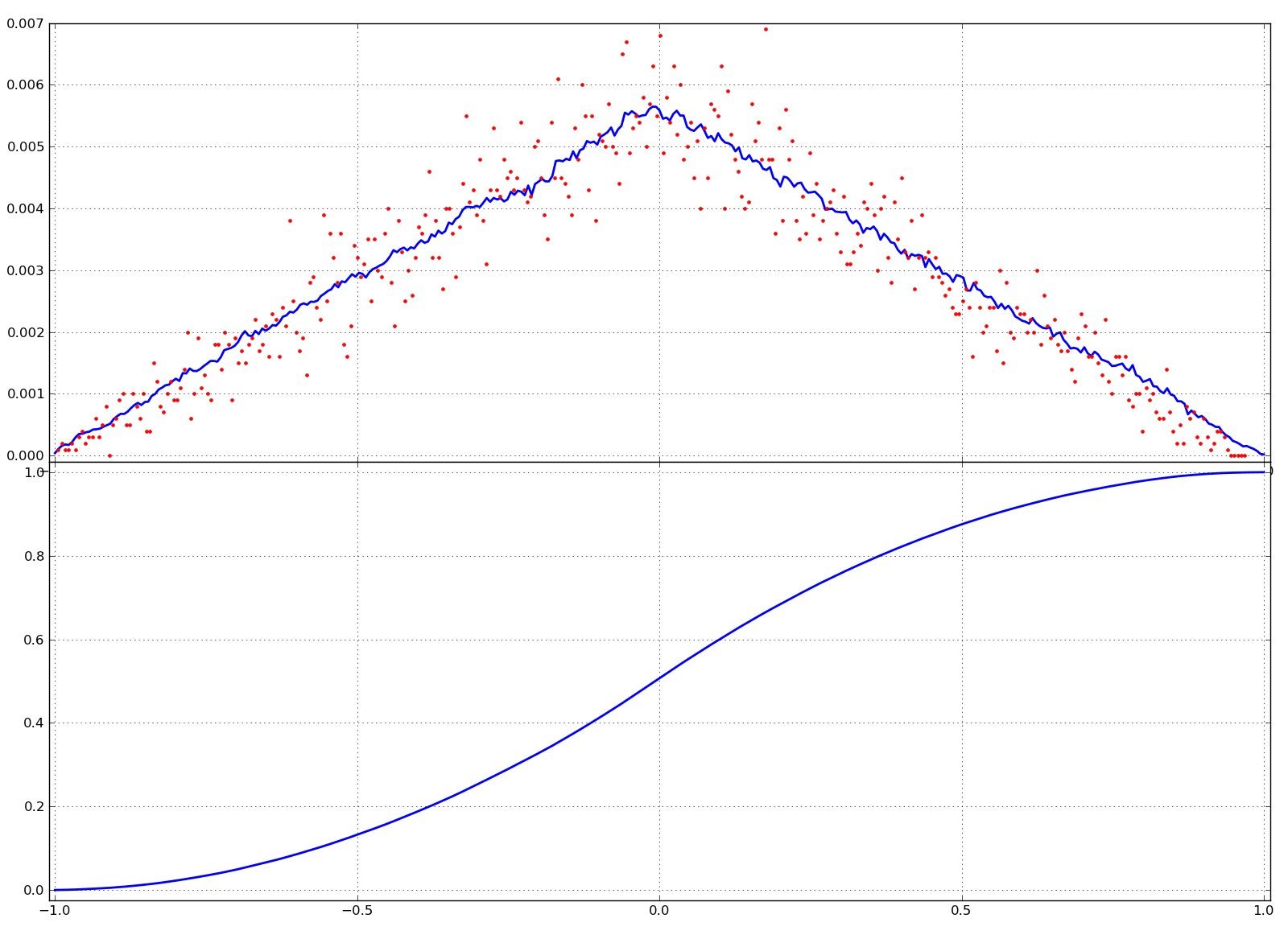
Where the upper part represents the
PDF
(blue line), which indeed looks quite triangular and the simulation (red dots), which reflects the triangular shape. Lower part represents theCDF
, which also looks to follow nicely the expectedS
-curve.