具有相同簇大小的 K 均值算法变体
我正在寻找最快的算法,用于按距离将地图上的点分组为大小相等的组。 k-means 聚类算法 看起来简单且有前途,但不能产生同等大小的组。
该算法是否有一种变体,或者是否有一种不同的算法可以允许所有集群的成员数量相等?
另请参阅:将 k 个簇中的 n 个点分组为相等尺寸
I'm looking for the fastest algorithm for grouping points on a map into equally sized groups, by distance. The k-means clustering algorithm looks straightforward and promising, but does not produce equally sized groups.
Is there a variation of this algorithm or a different one that allows for an equal count of members for all clusters?
如果你对这篇内容有疑问,欢迎到本站社区发帖提问 参与讨论,获取更多帮助,或者扫码二维码加入 Web 技术交流群。

绑定邮箱获取回复消息
由于您还没有绑定你的真实邮箱,如果其他用户或者作者回复了您的评论,将不能在第一时间通知您!
发布评论
评论(18)
这可能会起作用:应用 Lloyd 算法 来获取 k质心。按数组中相关簇的大小降序对质心进行排序。对于i = 1到k-1,将簇i中的数据点推送到任何其他质心j (i <j ≤ k) 到 j 并重新计算质心 i< /em> (但不要重新计算簇)直到簇大小达到n / k。
此后处理步骤的复杂度为 O(k² n lg n)。
This might do the trick: apply Lloyd's algorithm to get k centroids. Sort the centroids by descending size of their associated clusters in an array. For i = 1 through k-1, push the data points in cluster i with minimal distance to any other centroid j (i < j ≤ k) off to j and recompute the centroid i (but don't recompute the cluster) until the cluster size is n / k.
The complexity of this postprocessing step is O(k² n lg n).
以防万一有人想在这里复制并粘贴一个简短的函数 - 基本上运行 KMeans,然后在分配给集群的最大点(集群大小)的约束下找到点与集群的最小匹配
Just in case anyone wants to copy and paste a short function here you go - basically running KMeans then finding the minimal matching of points to clusters under the constraint of maximal points assigned to cluster (cluster size)
ELKI 数据挖掘框架有一个 等大小 k 均值教程。
这不是一个特别的算法,但它是一个足够简单的 k 均值变体,可以编写教程并教人们如何实现自己的聚类算法变体;显然,有些人确实需要他们的簇具有相同的大小,尽管 SSQ 质量会比常规 k 均值差。
在 ELKI 0.7.5 中,您可以选择此算法为
tutorial.clustering.SameSizeKMeansAlgorithm
。The ELKI data mining framework has a tutorial on equal-size k-means.
This is not a particulary good algorithm, but it's an easy enough k-means variation to write a tutorial for and teach people how to implement their own clustering algorithm variation; and apparently some people really need their clusters to have the same size, although the SSQ quality will be worse than with regular k-means.
In ELKI 0.7.5, you can select this algorithm as
tutorial.clustering.SameSizeKMeansAlgorithm
.一般来说,按照距离将地图上的点分成大小相等的组在理论上是一项不可能完成的任务。因为将点分组为大小相等的组与按距离将点分组为簇相冲突。
看这个情节:
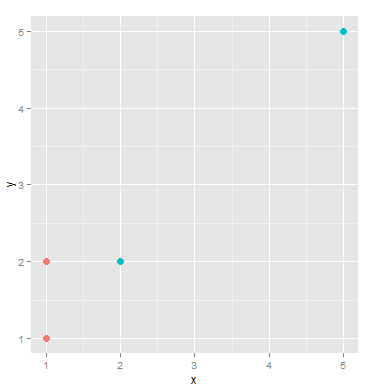
有四个点:
如果我们将这些点聚类为两个簇。显然,(A,B,C) 将是聚类 1,D 将是聚类 2。但如果我们需要相同大小的组,则 (A,B) 将是一个聚类,(C,D) 将是另一个聚类。这违反了聚类规则,因为 C 更接近 (A,B) 的中心,但它属于聚类 (C,D)。因此不能同时满足集群和同等大小的组的要求。
In general, grouping points on a map into equally sized groups, by distance is a impossible mission in theory. Because grouping points into equally sized groups is in conflict with grouping points in clusters by distance.
see this plot:
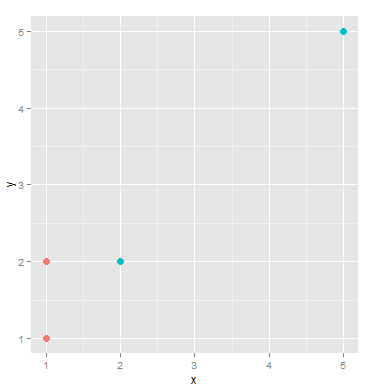
There are four points:
If we cluster these points into two cluster. Obviously, (A,B,C) will be cluster 1, D will be cluster 2. But if we need equally sized groups, (A,B) will be one cluster, (C,D) will be the other. This violates cluster rules because C is closer to center of (A,B) but it belongs to cluster (C,D). So requirement of cluster and equally sized groups can not be satisfied at the same time.
您可以将距离视为定义加权图。相当多的图划分算法明确地基于尝试将图顶点划分为两个大小相等的集合。例如,这出现在 Kernighan-Lin 算法 和 < a href="http://www.stanford.edu/~dgleich/demos/matlab/spectral/spectral.html#recursive" rel="noreferrer">使用拉普拉斯算子的谱图划分。要获得多个簇,可以递归地应用分区算法;在光谱图分区的链接上对此有一个很好的讨论。
You can view the distances as defining a weighted graph. Quite a few graph partitioning algorithms are explicitly based on trying to partition the graph vertices into two sets of equal size. This appears in, for example, the Kernighan-Lin algorithm and in spectral graph partitioning using the Laplacian. To get multiple clusters, you can recursively apply the partitioning algorithm; there's a nice discussion of this at the link on spectral graph partitioning.
尝试这个 k-means 变体:
初始化:
k
个中心,或者更好的是最后,您应该有一个分区,满足每个簇+-1相同数量的对象的要求(确保最后几个簇也有正确的数量)前
m
个簇应具有ceil
对象,其余的恰好是floor
对象。)迭代步骤:
必备条件:每个集群的列表,其中包含“交换建议”(希望位于不同集群中的对象)。
E 步骤:按照常规 k 均值计算更新的聚类中心
M 步骤:迭代所有点(可以是一个点,也可以是一批中的所有点)
计算最近的聚类中心到比当前聚类更接近的对象/所有聚类中心。如果是不同的簇:
簇大小保持不变(+- ceil/floor 差异),对象仅从一个簇移动聚类到另一个,只要它能导致估计的改进。因此它应该像 k 均值那样在某个点收敛。但它可能会慢一些(即更多迭代)。
我不知道这是否已经发布或实施过。这正是我会尝试的(如果我会尝试 k-means。有更好的聚类算法。)
Try this k-means variation:
Initialization:
k
centers from the dataset at random, or even better using kmeans++ strategyIn the end, you should have a paritioning that satisfies your requirements of the +-1 same number of objects per cluster (make sure the last few clusters also have the right number. The first
m
clusters should haveceil
objects, the remainder exactlyfloor
objects.)Iteration step:
Requisites: a list for each cluster with "swap proposals" (objects that would prefer to be in a different cluster).
E step: compute the updated cluster centers as in regular k-means
M step: Iterating through all points (either just one, or all in one batch)
Compute nearest cluster center to object / all cluster centers that are closer than the current clusters. If it is a different cluster:
The cluster sizes remain invariant (+- the ceil/floor difference), an objects are only moved from one cluster to another as long as it results in an improvement of the estimation. It should therefore converge at some point like k-means. It might be a bit slower (i.e. more iterations) though.
I do not know if this has been published or implemented before. It's just what I would try (if I would try k-means. there are much better clustering algorithms.)
在阅读了这个问题和几个类似的问题后,我使用 https://elki-project.github.io/tutorial/same-size_k_means 它利用 scikit-learn 的 K-Means 实现来实现大多数常见方法和熟悉的 API。
我的实现可以在这里找到:
https://github.com/ndanielsen/Same-Size-K-Means
聚类逻辑在此函数中找到:
_labels_inertia_precompute_dense()
After reading this question and several similar ones, I created a python implementation of the same-size k-means using the Elki tutorial on https://elki-project.github.io/tutorial/same-size_k_means which utilizes scikit-learn's K-Means implementation for most of the common methods and familiar API.
My implementation is found here:
https://github.com/ndanielsen/Same-Size-K-Means
The clustering logic is found in this function :
_labels_inertia_precompute_dense()
给定簇质心,有更干净的后处理。令
N
为项目数,K
为簇数,S = ceil(N/K)
最大簇大小。(item_id, cluster_id, distance)
(item_id, cluster_id, distance)
:cluster_id
中的元素数量超过S
则不执行任何操作item_id
添加到集群cluster_id
。这在 O(NK lg(N)) 中运行,应该给出与 @larsmans 解决方案相当的结果,并且更容易实现。在伪Python中
There is a cleaner post-processing, given cluster centroids. Let
N
be the number of items,K
the number of clusters andS = ceil(N/K)
maximum cluster size.(item_id, cluster_id, distance)
(item_id, cluster_id, distance)
in the sorted list of tuples:cluster_id
exceedsS
do nothingitem_id
to clustercluster_id
.This runs in O(NK lg(N)), should give comparable results to @larsmans solution and is easier to implement. In pseudo-python
考虑某种形式的递归贪婪合并——每个点都以单例簇开始,并重复合并最接近的两个点,这样合并不会超过最大值。尺寸。如果您别无选择,只能超过最大大小,则在本地重新集群。这是回溯层次聚类的一种形式:http://en.wikipedia.org/wiki/Hierarchical_clustering
Consider some form of recursive greedy merge -- each point begins as a singleton cluster and repeatedly merge the closest two such that such a merge doesn't exceed max. size. If you have no choice left but to exceed max size, then locally recluster. This is a form of backtracking hierarchical clustering: http://en.wikipedia.org/wiki/Hierarchical_clustering
最近我自己需要这个来处理不是很大的数据集。我的答案虽然运行时间比较长,但是保证收敛到局部最优。
Recently I needed this myself for a not very large dataset. My answer, although it has a relatively long running time, is guaranteed to converge to a local optimum.
等大小 k 均值是约束 k 均值过程的特殊情况,其中每个簇必须具有最小数量的点。这个问题可以表述为一个图问题,其中节点是要聚类的点,每个点到每个质心都有一条边,其中边权重是到质心的欧氏距离的平方。此处讨论:
Bradley PS、Bennett KP、Demiriz A (2000)、约束 K 均值聚类。微软研究院。
Python 实现可在此处获取。
Equal size k-means is a special case of a constrained k-means procedure where each cluster must have a minimum number of points. This problem can be formulated as a graph problem where the nodes are the points to be clustered, and each point has an edge to each centroid, where the edge weight is the squared euclidean distance to the centroid. It is discussed here:
Bradley PS, Bennett KP, Demiriz A (2000), Constrained K-Means Clustering. Microsoft Research.
A Python implementation is available here.
2012 年 1 月添加:
比后处理更好的是保持簇大小
与它们的生长大致相同。
例如,为每个 X 找到 3 个最近的中心,
然后将 X 添加到最好的那个
距离 - λ 簇大小。
如果 k 均值中的簇大小大致相等,则在 k 均值之后进行简单的贪婪后处理可能就足够了。
(这近似于 k-means 的 Npt x C 距离矩阵上的分配算法。)
可以迭代,
如果迭代改变中心很多,我会感到惊讶,
但这取决于™。
你的 Npoint Ncluster 和 Ndim 大约有多大?
Added Jan 2012:
Better than postprocessing would be to keep cluster sizes
roughly the same as they grow.
For example, find for each X the 3 nearest centres,
then add X to the one with the best
distance - λ clustersize.
A simple greedy postprocess after k-means may be good enough, if your clusters from k-means are roughly equal-sized.
(This approximates an assignment algorithm on the Npt x C distance matrix from k-means.)
One could iterate
I'd be surprised if iterations changed the centres much,
but it'll depend ™.
About how big are your Npoint Ncluster and Ndim ?
我将答案中提出的大部分算法添加到存储库 https://github.com/brand17/clusters_equal_size< /a>.
最有效的是得票最多的答案。
我开发了一些其他算法(得票最多的仍然是最好的):
我修改了迭代交换提案 - 通过识别和消除直接循环而不是仅仅交换(它提高了一点效率)
我还开发了以下算法:迭代最近的质心对并通过有效移动 Voronoi 图边界来交换它们之间的点,以便点数相差不超过 1。
I added a majority of the algorithms presented in the answers to the repository https://github.com/brand17/clusters_equal_size.
The most efficient is the most voted answer.
I developed a couple of other algorithms (the most voted is still the best):
I modified iterating swap proposals - by identifying and eliminating direct cycles rather than just swapping (it improves efficiency a bit)
I also developed the following algorithm: iterating pairs of closest centroids and swapping points between them by efficiently moving Voronoi diagram border so that the number of points differs by no more than one.
另请查看 Kd 树,它对数据进行分区,直到每个分区的成员小于 BUCKET_SIZE(算法的输入)。
这不会强制存储桶/分区的大小完全相同,但它们都会小于 BUCKET_SIZE。
Also look at K-d tree which partitions the data until each partitions' members are less than a BUCKET_SIZE which is an input to the algorithm.
This doesn't force the buckets/partitions to be exactly the same size but they'll be all less than the BUCKET_SIZE.
我可以谦虚地建议您尝试这个项目 ekmeans。
它还处于实验阶段,因此请注意已知错误。
May I humbly suggest that you try this project ekmeans.
It is yet experimental, so just be aware of the known bugs.
我也一直在纠结如何解决这个问题。然而,我意识到我一直使用了错误的关键字。如果您希望点结果成员的数量相同,那么您正在进行分组,而不是聚类。我终于能够使用简单的 python 脚本和 postgis 查询解决问题。
例如,我有一个名为 tb_points 的表,其中有 4000 个坐标点,您想将其分为 10 个相同大小的组,每个组包含 400 个坐标点。这是表结构的示例
那么您需要做的是:
这是 python 中的实现:
希望它有帮助
I've been struggling on how to solve this question too. However, I realize that I have used the wrong keyword all this time. If you want the number of point result member to be same size, you are doing a grouping, not clustering anymore. I finally able to solve the problem using simple python script and postgis query.
For example, I have a table called tb_points which has 4000 coordinate point, and you want to divide it into 10 same size group, which will contain 400 coordinate point each. Here is the example of the table structure
Then what you need to do are:
This is the implementation in python:
Hope it helps
在簇分配过程中,我们还可以在距离上添加“频率惩罚”,这使得高频簇更难获得额外的分数。这在“频率敏感竞争学习”中进行了描述
高维超球面的平衡聚类 - Arindam Banerjee 和 Joydeep Ghosh - IEEE Transactions on Neural Networks"
http://www.ideal.ece.utexas.edu/papers/arindam04tnn.pdf
他们还有在线/流媒体版本。
During cluster assignment, one can also add to the distance a 'frequency penalty', which makes it harder for high-frequency clusters to get additional points. This is described in "Frequency Sensitive Competitive Learning for
Balanced Clustering on High-dimensional Hyperspheres - Arindam Banerjee and Joydeep Ghosh - IEEE Transactions on Neural Networks"
http://www.ideal.ece.utexas.edu/papers/arindam04tnn.pdf
They also have an online/streaming version.
您想要查看空间填充曲线,例如 z 曲线或希尔伯特曲线。您可以考虑一条空间填充曲线,将二维问题简化为一维问题。尽管 sfc 索引只是二维数据的重新排序,而不是数据的完美聚类,但当解决方案不能满足完美聚类并且必须相当快地计算时,它可能很有用。
You want to take a look into space-filling-curve, for example a z-curve or a hilbert-curve. You can think of a space-filling-curve to reduce the 2-Dimensional problem to a 1-Dimensional problem. Although the sfc index is only a reorder of the 2-Dimensional data and not a perfect clustering of the data it can be useful when the solution has not to satisfied a perfect cluster and has to be compute fairly fast.